
The highest-mass subnuclear particle ever observed used to the the top quark. Measured for the...
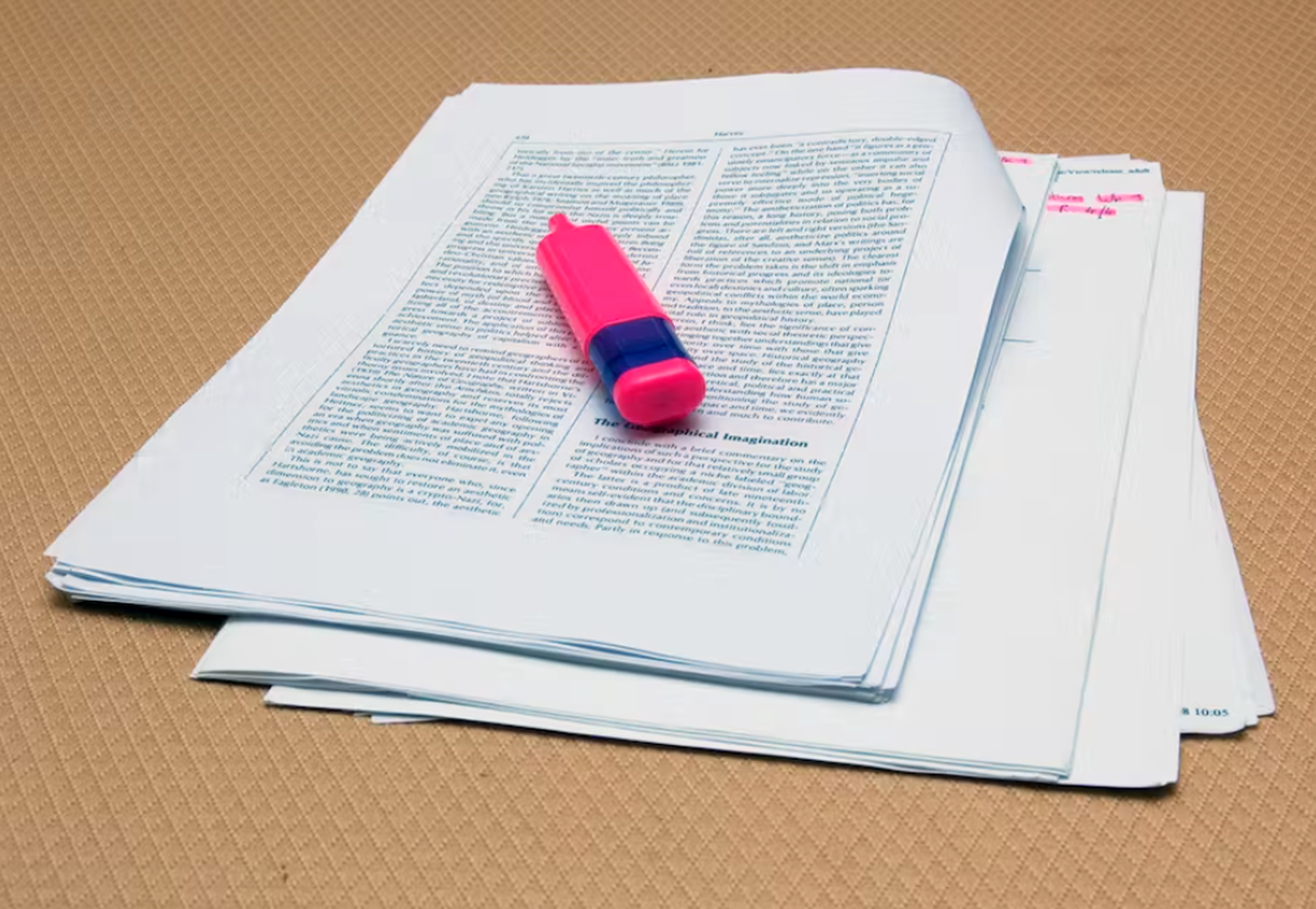
In a world where misinformation, voluntary or accidental, reigns supreme; in a world where lies...
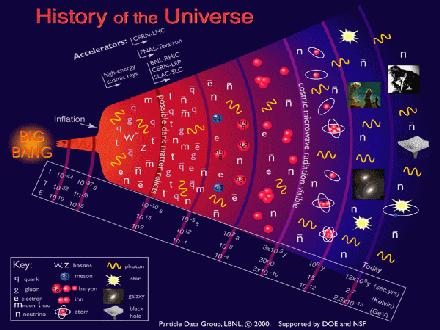
In the past few years my activities on this site - but I would say more in general, as the same...
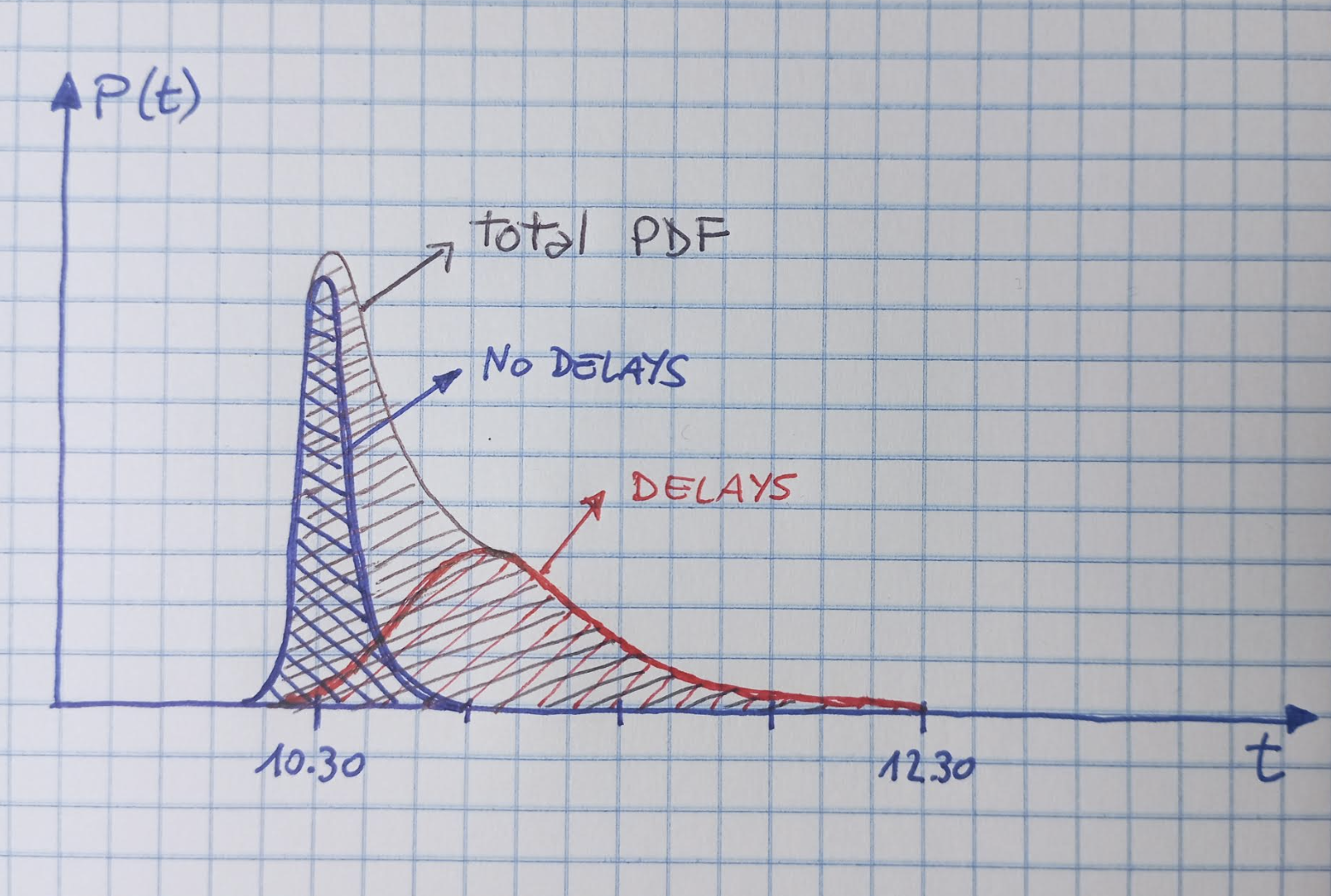
Perhaps the most important thing to get right from the start, in most statistical problems, is...