The heart disease drug perhexiline is one of 101 compounds predicted to prevent cancer growth in most patients suffering from our most common liver cancer, HCC. This is an outcome from a novel simulation-based approach using personal sets of proteins of six HCC patients.
"This is the first time personalized models have been used to find and evaluate new potential drugs," says Professor Jens Nielsen at Chalmers University of Technology.
--
Our most common liver cancer, Hepatocellular carcinoma, HCC, causes more than half a million deaths worldwide every year. If the cancer cannot be surgically removed the disease is usually deadly within 3-6 months. Only 30 percent of the patients respond to the best existing drug, sorenafib. This new research now identifies 101 drug candidates predicted to prevent the cancer growth in all six studied patients, which raises hope of developing a drug helping all HCC-patients.
Cancer cells modify their metabolism in order to breed. To understand these diverse mechanisms, what metabolic enzymes are involved, when and how, has been a major focus in medicine in order to identify novel drug targets. However, this is quite a challenging task, since HCC not only involves a large number of interplays between different biological pathways, but also significant individual variations.
Finding the candidates
Average models don't give sufficiently good answers. To take the individual variations into account the researchers generated personalized proteomics data for HCC patients using the antibodies produced in the Human Protein Atlas project (read more below). The researchers then generated individual computer models for six HCC patients based on their entire, personal set of proteins and a generic map of human metabolism, which had been produced in an earlier project.
"I am excited to see how we have managed to successfully transfer our modelling approaches on yeast to study cancer metabolism," says Dr Rasmus Ågren, shared first author of the paper.
The six personal models were then used to find potential new anticancer drugs. One of the most common types of anticancer drugs is so called antimetabolites. Antimetabolites prevent the use of one or more metabolites (the small molecules that act and are produced, and whose interplay together constitute a full metabolism) by stopping the catalyzing enzymes. By simulating the effect of all possible antimetabolites – more than 3000 compounds – the computer generated potential anti-cancer drugs which could be effective in inhibiting tumor growth.
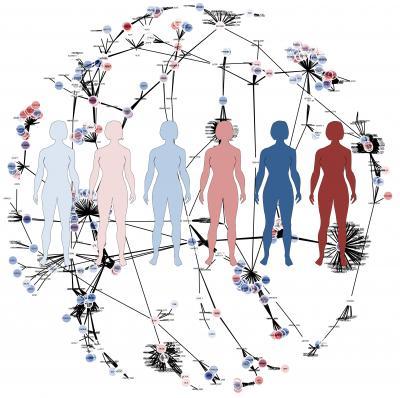
This is an illustration of the genome-scale metabolic models.
(Photo Credit: Martina Butorac)
Furthermore, the researchers simulated the effect of these antimetabolites on 83 major healthy cell types in human body to predict their toxic effects. This led to the identification of 101 antimetabolites which were predicted to prevent cancer growth in all six studied HCC patients, whereas 46 antimetabolites inhibited tumor growth only in one or a few of the patients. All 147 were predicted to not be overly toxic to healthy cells.
Clinical tests
The general validity of the approach can be extended by running personalized models for more patients.
"With this approach we can find and evaluate new potential drugs, some that could be used for general treatment of HCC, and others that are highly specific for each HCC patient. We can also predict false positive drug targets that would not be effective in all patients. This would lead to more targeted and efficient cancer treatment," says Dr Adil Mardinoglu, shared first author of the paper.
One of the antimetabolites was tested in vitro on a liver cancer cell line. The tested compound, perhexiline, had an effect on viability comparable to sorafenib, demonstrating the predictive power of the computer models. Perhexiline is a drug approved for heart disease, and the potential to use it for HCC is of course interesting. As a continuation of this study, perhexiline will be tested on other cancer cell lines, since the researchers believe it may inhibit growth in other types of cancer as well. The initial experiments look very promising.
Read the article in Molecular Systems Biology: Identification of anticancer drugs for hepatocellular carcinoma through personalized genome-scale metabolic modeling
FACTS
Mass production of personalized models with new algorithm
Tinit, a new algorithm for automatic reconstruction of personalized models, was recently developed at Chalmers University of Technology, branded as tINIT. Compared to previous algorithms it allows reconstruction of functional and simulation ready models. All the tools for have been made publically available in the so called Raven toolbox.
Proteomics data in Human Protein Atlas
All of the models were generated based on the proteomics data generated in Human Protein Atlas, HPA. The Human Protein Atlas project is a world leading effort to systematically explore the human proteome in 46 different types of normal tissue and 216 different cancer tissues representing the 20 most common forms of human cancer. The atlas was recently used to generate a virtual map of human metabolism, in a collaborative project between Chalmers University of Technology and researchers at SciLifeLab. This virtual map of human cell metabolism has the most comprehensive list of the entire set of known biochemical reactions which occurs in human body. The map contains more than 8,000 reactions and 3,765 associated genes, and it represents a great resource for systems biologists working in human disorders related to metabolism.
Read the article in Molecular & Cellular Proteomics: Analysis of the Human Tissue-specific Expression by Genome-wide Integration of Transcriptomics and Antibody-based Proteomics
Drug targets and biomarkers also for other liver conditions
The same type of modelling (however using an average model) has previously been used to investigate the most common liver disease, non-alcoholic fatty liver disease. This resulted in predictions of new drug targets, new biomarkers for diagnosis, as well as suggestions for dietary interventions.
Read more about that study in the Chalmers' news item Computer model takes us closer to treatment of our most common liver disease, or the Nature Communications article Genome-scale metabolic modelling of hepatocytes reveals serine deficiency in patients with non-alcoholic fatty liver disease.
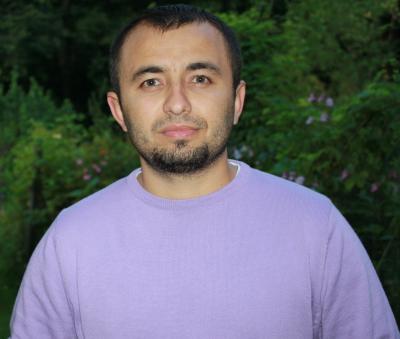
Dr. Adil Mardinoglu is with the Systems Biology group at Chalmers University of Technology.
(Photo Credit: Iryna Belova)
Comments